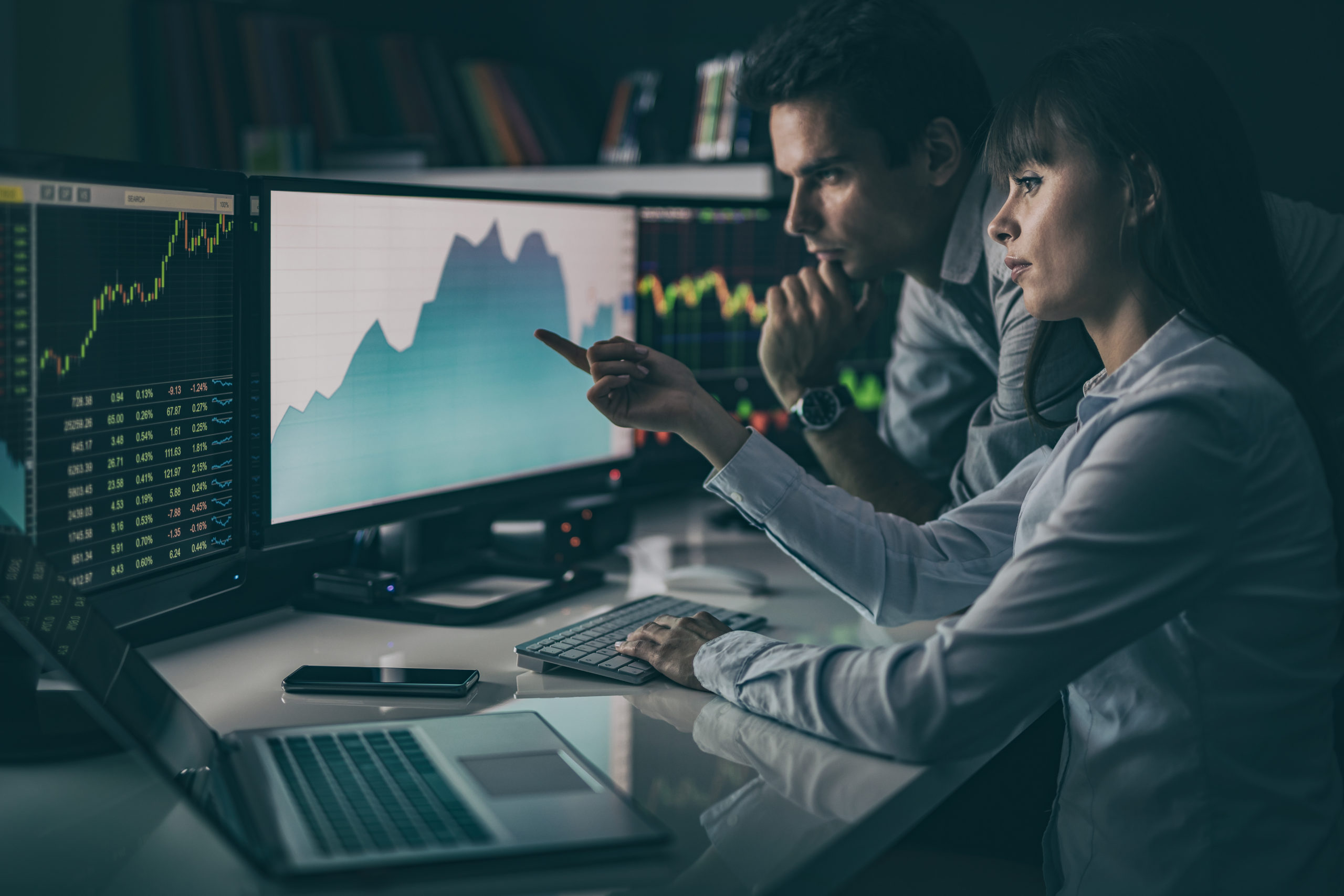
Recent attempts to clarify the EPO’s approach to examining machine learning applications have arguably been missing an important factor… a body of case law on which to base the guidance. The section on AI and machine learning in the EPO guidelines for examination lists two narrow and specific cases in the classification section but no law relating to the broader principles. While machine learning applications have been filed for many (many) years at the EPO, it is only recently that filing numbers have significantly increased to the point where ML (and AI) is the current ‘hot topic’. This lack of significant case law has led to a lot of uncertainty for applicants but also a big public relations push by the EPO to spread the message to applicants about how the patentability of machine learning based inventions should be assessed.
While not explicitly related to machine learning, the principles set out in the most recent decision of the Enlarged Board of Appeal in respect of simulations (G1/19) are likely to provide useful guidance to applicants and examiners in how to approach certain categories of machine learning inventions at the EPO. The guidance given in G1/19 will support the EPO’s guidelines with analogous case law where in the past some applicants might have felt that the EPO’s approach lacked sufficient basis.
The similarities between simulations and machine learning algorithms are obvious. Even the language used is the same: simulations create models which model a particular phenomena; machine learning creates models to make predictions. The mathematical techniques used are also often similar.
During the proceedings at the Enlarged Board, this similarity was mentioned throughout. Indeed it was discussed in G1/19 that machine learning could be viewed as ‘empirical simulation’ in which the underlying equations governing what is being simulated might not be known, unlike a traditional simulation which is analytical in the sense that the simulated system evolves according to a set of well-defined equations.
Informally it was always a concern to practitioners — what would the impact of this decision be on machine learning? Would the nature of the questions have unintended consequences? The answer thankfully is no, but we do now have clarification and analogous law we can use in other fields.
The Enlarged Board were considering in particular whether or not a computer-implemented simulation of a technical system solves a technical problem by producing a technical effect which goes beyond the simulation’s implementation on a computer (effectively, are simulations patentable at the EPO?).
The answer was that a model itself is not patentable (it is not technical). What makes a simulation patentable is the benefit that simulation provides or the outcome of the simulation. For example, whether or not the simulation is a better simulation (e.g. improved use of computer resources) or whether or not the simulation has an impact on a technical process (e.g. using a weather forecast simulation to automatically open or close shutters on a building).
Looking at the conclusions of G1/19 we can see that there is useful guidance here:
“…numerical simulations ML algorithms may be patentable if an inventive step can be based on features contributing to the technical character of the claimed simulation ML method”
“However, they may contribute to technicality if, for example, they are a reason for adapting the computer or its functioning, or if they form the basis for a further technical use of the outcomes of the simulation ML algorithm (e.g. a use having an impact on physical reality)”
The conclusions of G1/19 will most likely be equally applicable to many machine learning inventions. Machine learning algorithms and models will not themselves be patentable, they will likely be considered to be abstract mathematical entities only. However, machine learning may be patentable if the benefit provided is technical. Machine learning isn’t likely to be patentable just because it is learning (or predicting) something technical. If the machine learning process is replicating a human activity then it will be unlikely to lead to a patentable invention.
To be patentable, the machine learning invention should have a technical effect such as improving how the algorithm functions on the computer, making the computer use resources more effectively or controlling an external process; the latter being at least implicitly referenced in the claim.
If you would like information on how to protect your company’s innovation or have any questions in relation to this article, please find my contact details on my website profile here or contact us at gje@gje.com.
GJE Review: Simulations
GJE’s computer technology team have put together a comprehensive collection of content designed to equip your business with insight into simulations and the role intellectual property has in its development. To view the full collection, click here.